VISSECT - Visual Segmentation and Labeling of Multivariate Time Series
This project's goal is to develop new approaches for visually and interactively analyzing multivariate time series through segmentation and labeling. The ground-breaking novel idea is to combine the algorithm selection, the adequate parametrization, and the visualization and exploration of diverse types of uncertainty about the results.
- Silvia Miksch
- Theresia Gschwandtner
- Markus Bögl
- Christian Bors
D-A-CH - Austrian Science Fund (FWF), grant I 2850-N31.
The overarching objective of this research project is the combination of the three most relevant steps in the process of segmenting and labeling multivariate time series: algorithm selection, parametrization, and uncertainty analysis. Each of these steps is a research problem on its own, and we will contribute novel solutions to all of them. However, only the combination of the different aspects will comprehensively address the important challenge of visual analytics as identified by Jarke van Wijk: "Which model? Which parametrization? And which feature of the data?" In the following we will describe the underlying research questions for the individual aspects with regard to the envisaged interconnections.
Algorithm selection: Segmentation and labeling algorithms divide multivariate time series into smaller segments and label these segments accordingly. The effect of a particular algorithm on a particular data set is not easily predictable and thus finding the best algorithm in a great diversity of existing algorithms is highly demanding.
Parametrization: The effect of different parametrizations on the segmentation process is not easy to understand, and thus finding the best setting is challenging. Particularly in the case of large parameter spaces (many parameters and large value ranges) one typically has to rely on trial and error to find adequate configurations. So, the main goal of this objective is to develop sophisticated visual analytics techniques for a systematic analysis of the parameter spaces.
Uncertainty: The generated segmentation and labeling results may comprise different kinds of uncertainty at different levels. These stem from the selection of algorithms, parameters, and the calculation of multiple competing results.
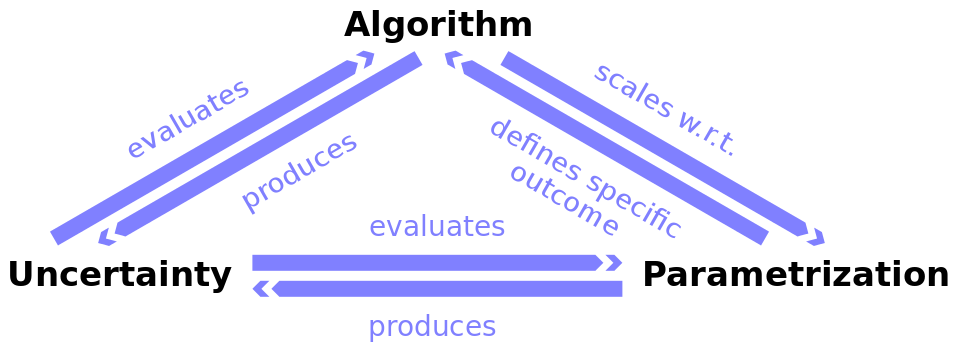